Researchers in SPHTM are at the forefront of efforts to bring AI and machine learning to the examination and identification of solutions to critical public health problems across the globe.
Diabetes
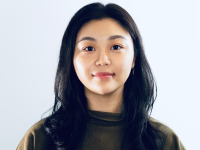
In the U.S., approximately 38.4 million Americans, or 11.6% of the population, have diabetes.3 Faculty members Dr. Lizheng Shi and Dr. Yilu Lin (HPAM) conducted groundbreaking research on predicting diabetes complications using modern risk engines and machine learning.4 Their study highlights the BRAVO model, an advanced AI-driven tool capable of accurately forecasting diabetes-related outcomes and assessing long-term cost-effectiveness of interventions. This innovative approach aims to improve patient care by enabling personalized treatment plans and effective risk stratification.
Sickle-Cell Anemia
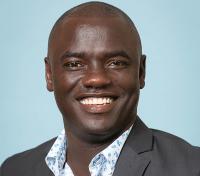
Sickle-cell anemia is a genetic blood disorder that predominantly affects people of African descent, including approximately one in every 365 Black or African American (Black) newborns.5 Department of Biostatistics and Data Science faculty member Dr. Samuel Kakraba was involved in a ground-breaking study using a machine learning model to study how changes in protein structures can lead to diseases like sickle cell anemia. Using machine learning, the research team created a detailed map of interactions between parts of a protein, allowing the researchers to simulate how a single mutation can impact the protein’s function. The machine-learning algorithm used a dendogram, an unsupervised learning method, to visualize these changes and enhance the capacity to identify different patterns of the disease. This work was instrumental in improving understanding of protein-related diseases and potential therapeutic interventions.6
Gender-based Violence
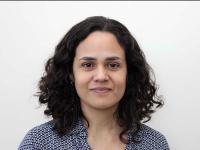
According to the World Health Organization, more than one in three women of reproductive age in Sub-Saharan Africa, or approximately 83 million women, have been victims of physical or sexual gender-based violence (IPV) in their lifetimes.1 A pioneering study by Dr. Martha Silva (IHSD), PhD candidate Udochisom Anaba (IHSD), and colleagues in Washington, DC and Nigeria,2 funded under the USAID Breakthrough Research project, utilized artificial intelligence methods in social listening to online conversations related to gender-based violence in Nigeria. This innovative research, revealed a significant increase in discussions around sexual consent and a substantial decline in slut-shaming over five years, highlighting the impact of social movements and popular culture on public discourse. The study underscored the importance of leveraging digital platforms and influencers to foster gender equity and combat gender-based violence.
Youth Sexual and Reproductive Health
As part of a multi-method evaluation of the Merci Mon Héros (MMH) campaign, which aimed to improve the normative environment in support of intergenerational communication about sexual and reproductive health topics in western Africa, Dr. Martha Silva (IHSD) and colleagues utilized social media monitoring and social listening approaches to provide real-time programmatic feedback.7 AI-powered analyses provided significant insights that led to adaptive shifts in the campaign. For instance, recognizing that the Facebook campaign followers were primarily male prompted the team to tailor calls to action to engage the male audience and adjust marketing strategies to attract more female followers. They also found that shorter videos were more likely to be viewed in their entirety, leading to the creation of concise video content and teaser screenshots to enhance engagement. Additionally, by addressing negative sentiments associated with beliefs against premarital sex, the campaign included content featuring religious leaders who promoted open discussions with young people about reproductive health. The findings demonstrate how artificial intelligence and social media platforms can be powerful tools for informing and engaging diverse audiences on public health issues, monitoring opinions and behaviors, and effectively promoting public health initiatives in Sub-Saharan Africa.
Violence Prevention and Narratives Surrounding Violence
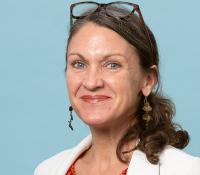
Dr. Kat Theall, Dr. Samantha Francois, and SBPS PhD student Simone Skeen of the Violence Prevention Institute (VPI) and its Center for Youth Equity (CYE) are currently utilizing Machine Learning (ML) and Natural Language Processing (NLP) to analyze social media data to characterize narratives surrounding violence, and firearm violence in particular, and to examine how well narratives discuss evidence-based prevention approaches. VPI students have also utilized ML-NLP to characterize suicidal ideation in social media data and the impact of U.S. policies against bodily autonomy on state-level trends in suicidal ideation. Additional approaches are being investigated to utilize AI for improved hospital electronic medical record classification for violence and injury events.
AI-Powered Systematic Literature Review
A task force of Tulane University and the University of Mississippi Medical Center has evaluated the process and effectiveness of using large language model (LLM) to perform a systematic literature review of economic evaluation articles. The work has demonstrated LLM-powered SLR platform's reliability, accuracy, and efficiency in data extraction. However, we also identify the challenges of using AI tools (e.g., ChatGPT) for selecting relevant articles.
Generative AI in Public Health Research
Students and faculty in the Department of Health Policy and Management are currently evaluating the utility of generative AI tools (e.g., ChatGPT, Gemini) in public health research, with initial findings highlighting both strengths and limitations. While these tools are not suitable for conducting systematic literature reviews due to their lack of rigor and comprehensiveness, they are effective in drafting initial code for econometric research methods in various statistical programming languages, provided the user has a strong foundational knowledge of the language. Additionally, the researchers also found that generative AI cannot reliably produce complete lists of ICD codes for specific diseases but can offer a helpful starting point to guide researchers toward relevant sections of ICD codebooks. These results suggest that generative AI can serve as a complementary tool for researchers.
1World Health Organization. (2021). Violence against women prevalence estimates, 2018. World Health Organization. Available from https://apps.who.int/iris/handle/10665/341337
2Silva M, Anaba U, Jani Tulsani N, Sripad P, Walker J, Aisiri A. (2023) Gender-Based Violence Narratives in Internet-Based Conversations in Nigeria: Social Listening Study. J Med Internet Res. 2023 Sep 15;25:e46814. doi: 10.2196/46814. PubMed PMID: 37713260; PubMed Central PMCID: PMC10541644.
3American Diabetes Association. (2023). Statistics about diabetes. Diabetes.org. https://diabetes.org/about-diabetes/statistics/about-diabetes
4Shao H, Shi L, Lin Y, Fonseca V. (2022) Using modern risk engines and machine learning/artificial intelligence to predict diabetes complications: A focus on the BRAVO model. J Diabetes Complications. 2022 Nov;36(11):108316. doi: 10.1016/j.jdiacomp.2022.108316. Epub 2022 Oct 3. Review. PubMed PMID: 36201893.
5 Kayle M, Blewer AL, Pan W, et al. Birth Prevalence of Sickle Cell Disease and County-Level Social Vulnerability — Sickle Cell Data Collection Program, 11 States, 2016–2020. MMWR Morb Mortal Wkly Rep 2024;73:248–254. DOI: http://dx.doi.org/10.15585/mmwr.mm7312a1
6 Edem K. Netsey, Samuel Kakraba, Samuel M Naandam, Aayire C. Yadem. (2022) A Mathematical Graph-Theoretic Model of Single Point Mutations Associated with Sickle Cell Anemia, Journal of Advances in Biotechnology (JBT), 9: 1-14
7 Silva M, Walker J, Portillo E, Dougherty L. (2022) Strengthening the Merci Mon Héros Campaign Through Adaptive Management: Application of Social Listening Methodology, JMIR Public Health Surveill 2022;8(6):e35663, doi: 10.2196/35663